The report from an NHMRC-organised workshop that brought together experts to discuss the opportunities and risks from the use of AI in NHMRC and the Department of Health and Aged Care Health and Medical Research Office (HMRO)-funded health and medical research, and its translation. This was to ensure Australia is well positioned to make the best use of the technologies to improve human health.
Publication Data
Background
NHMRC’s Council asked the Office of NHMRC (ONHMRC) to consider opportunities and risks from the use of artificial intelligence (AI) in NHMRC-funded research and translation.
In NHMRC’s Corporate Plan 2023-24, the health priority Identifying emerging technologies in health and medical research and in health care has a key action to Recognise the growing importance of artificial intelligence and consider its impact on NHMRC grant processes and opportunities for improving health outcomes.
ONHMRC organised a workshop on 29 May 2024 with experts, whose expertise covered technical, ethical, social and implementation applications for AI, and government officials from NHMRC, the Department of Health and Aged Care, the Australian Research Council and the Department of Education.
The aim of the workshop was to bring together experts to discuss the opportunities and risks from the use of AI in NHMRC and HMRO-funded health and medical research, and translation of this research. This was to ensure Australia is well positioned to make the best use of the technologies to improve human health.
The objectives of the workshop were to obtain advice from experts on where NHMRC/HMRO can focus for:
- Investment - funding high-quality health and medical research and building research capability for using AI
- Translation - supporting the translation of health and medical research using AI into better health outcomes
- Integrity and Ethics - promoting the highest standards of ethics and integrity in health and medical research using AI.
The following questions were posed to workshop participants:
- What are Australia’s research workforce strengths in using AI in health and medical research? What can NHMRC/HMRO do to build on this?
- What are the gaps or barriers to developing AI capability in research in Australia? What can NHMRCC/HMRO do to cover or address this?
- What could NHMRC/HMRO do to improve translation of health and medical research using AI?
- How can NHMRC/HMRO policies help to achieve value from AI research?
- What NHMRC-owned documents might need some extra guidance or policy about use of AI? for example, Code for the Responsible Conduct of Research, National Statement on Ethical Conduct in Human Research.
- Do we need a Guide for AI under the Code? What should be covered by this guidance?
- Do we need stand-alone ethics guidance for the use of AI, separate to the National Statement on Ethical Conduct in Human Research? What should be covered by this guidance?
- What is our aspiration/vision of AI in health and medical research? At the same time, which should NHMRC/HMRO do next week?
- What externally developed resources can NHMRC/HMRO use to support policies or advice on AI use?
The background information provided to workshop participants is at Appendix A.
NHMRC participated in the Australian Public Service trial of Copilot for Microsoft 365. The workshop was recorded for internal NHMRC note-taking purposes. Copilot was used to assist with transcribing and summarising the discussion. Electronic note boards (EasyRetro) were used during the workshop for participants to provide written input.
Workshop outcomes
NHMRC’s CEO Professor Wesselingh outlined NHMRC and HMRO’s previous investments in research involving or related to AI (Appendix B). He noted that NHMRC’s focus was on investing in high-quality research, building research capability for AI, and promoting the highest standards of ethics and integrity.
Professor Enrico Coeira spoke about AI in health research and its impact on the grant application and assessment process. He also discussed the broader question of how to support health research in AI. Professor Coeira suggested benchmarking NHMRC’s funding for AI research internationally, to consider large national capability lifting entities to fund AI research and including people with AI expertise on peer review panels.
Participants stated that AI is already being used in research and healthcare, and that the challenges are present and urgent. They noted that the types of AI vary and examples include AI as a tool like statistics, an AI intervention such as a chatbot for mental health, AI analysis of medical imaging, AI to build predictive models, AI for grant writing, AI to assist NHMRC internal processes in managing grant applications, AI to assist with peer review, and basic application of AI in drug discovery.
'The use of Large Language Models through tools such as ChatGPT is new and we need research into how to use that safely and effectively.'
They advised that AI should be unpacked into its component parts, and not treated as a monolithic entity. Different AI models, modalities and techniques are appropriate for solving different types of health problems and health system problems. They advised that unpacking the ‘black box of AI’ could lead to a number of transitional and policy activities for NHMRC and HMRO.
Australia’s research workforce strengths in using AI in health and medical research
Workshop participants identified the following areas of research workforce strength in using AI:
- image processing and interpretation for example, breast cancer screening, skin cancer screening, eye disease
- development of AI algorithms for analytics, imaging, genomics clinical data
- significant AI skills in research engineering, IT and computer science departments
- use of machine learning and AI to uncover insights from genomics
- expertise in Human-Computer Interaction and Information Systems that can be leveraged to support evaluation of real-world deployment of AI into health settings/applications
- research in the safety of AI in healthcare
- improvements in neurology by enhancing diagnostic accuracy, personalizing treatment, improving patient care, and advancing our understanding of neurological disorders.
Gaps or barriers to developing AI capability in research in Australia
Participants discussed a range of challenges and barriers that hinder the development and translation of AI in health and medical research. A few participants said that while there have been advances in some areas, such as diagnostics and workforce logistics, these have not been seen at all in other parts of healthcare.
Capability
Participants identified several gaps and barriers specific to capability, including:
- the need to balance the use of AI as a tool versus making it the centrepiece of the research
- a lack of multidisciplinary collaboration:
- the need to combine clinical, technical and ethical perspectives on use of AI
- the niche skills required in AI and the challenges of those experts working on multiple grants in the same round, which limits their ability to contribute to multiple projects
- the shortage of data scientists in the health sector and the competition with other industries
- AI literacy and skills in the health and medical research workforce
- a need for robust evaluation frameworks for establishing evidence around use/translation of AI, such as whether AI is giving insight into disease mechanisms and changing clinical decision making.
Data
Several gaps and barriers specific to data were identified. These were:
- challenges of timely access to data for researchers
- lack of data to train AI in some areas for example, digital health records
- complexities of data sharing in the context of patients with rare diseases
- AI standards and their interaction with healthcare
- data governance
- health informatics basics such as data standards, health data semantics and data quality that are critical for robustness and transportability of any methods/models built on top of health data.
'Anything that's data-driven is sensitive to the structure and the documentation practice, the data entry practices, the data semantics. There are very, very few examples of tools that have been developed in one healthcare setting with one set of data and successfully been transported into a different healthcare setting with a different data environment, a different population environment.'
Investment in health research using AI
'Why are we going to fund AI? Is it because we want to uplift our national capability? Is it because we specifically want to see commercial innovation and economic benefit? Is it because we want to improve our outcomes? Is it that we want a resilient health system? We probably want all of those things, but probably can't necessarily prioritise them all.'
Several of the participants commented on a perceived funding gap between NHMRC and ARC.
There was strong agreement that it is very important to fund multidisciplinary teams for health research using AI. Types of people who could be involved were identified:
- clinicians
- machine learning and AI experts
- health communications experts
- ethicists, bioethicists, philosophers
- end users and consumers, diverse groups of consumers
- underserved/minority groups, people from minority backgrounds, people with rare diseases
- implementation scientists.
'It's super important to involve clinical / medical domain experts from the very start to build trust and to ensure that the "right" problem is being solved.'
Translation of health research using AI
The following were identified as important to facilitating translation of health research using AI:
- public engagement and trust in AI
- including implementation scientists in multidisciplinary teams, to ensure that AI-based interventions are integrated into clinical workflows
- having work packages within projects that focus on translation of the research
- including the costs of regulatory approval through the Therapeutics Goods Administration in research budgeting
- addressing the willingness of clinicians to use AI-assisted decision support tools and patient preferences of having people making decisions for their care.
'Doctors might hesitate to use AI if they aren't sure who's responsible for its decisions. But if they ignore AI advice and something goes wrong, they could be blamed. This uncertainty may make doctors wary of using new technology.'
Ethics and Integrity
Participants raised some ethical and integrity challenges for AI research, such as the skills of ethics committees to assess applications involving AI, the standard of informed consent, the secondary use of data, privacy and security of data, the transparency and explainability of AI, and potential bias and discrimination in AI.
'You want the current committees to be skilled up enough to deal with the AI coming their way and then have access to expertise and bring those people into the room to explain what's going on.'
Participants identified a need for ethics committee members to better understand the risks and benefits of AI when assessing ethics applications. There are specific challenges around how to interpret key principles in the context of AI research, for example identifiability, potential for harm, dual use and bias. They also questioned whether AI development projects are being submitted for ethics review and whether/when they should be.
Other areas identified were:
- the importance of data privacy in training large models and the need for guidance on what level of privacy is needed
- the importance of trust in the use of AI from the perspective of consumers and clinicians, as well as researchers and ethics committees
- data accessibility and governance issues such as access to high quality datasets for training, data sharing, data linkage, data standards, governance frameworks for secondary use of health data
- the need for transparency and accountability in the use of AI, particularly in the context of informed consent and data security
- assessing centrally commonly-used AI tools and models and providing guidance to researchers on how compliant they are with Australia's research ethics and integrity guidelines
- the need to address the health equity and performance issues that arise from differences between data used to train AI versus the population intended for the AI.
Participants agreed that there was no need for a new standalone resource for ethics and integrity guidance for researchers and ethics committees to draw from pertaining to AI. They advised that current guidance could have extra information about issues specific to AI research.
'Not standalone, but specific. There are increasingly complex issues that are difficult for HRECs [Human Research Ethics Committees] to interpret without specialist resources and support.'
Workshop participant suggestions for NHMRC and HMRO
The participants were asked to give suggestions that NHMRC and HMRO could take. Their recommendations are summarised below.
Suggestions specific to AI
- Unpack the ‘black box of AI’.
- Provide guidance and education to clinicians and the public on the use and evaluation of AI in research and healthcare, in order to increase skills and trust.
- Use NHMRC/HMRO's social license to develop a campaign or a statement to increase trust in AI or in sharing of health data for research purposes. Developing some compelling stories or case-studies of improved health outcomes using AI.
- Benchmark and measure the impact and benefits of AI in health and compare with international best practices.
- Consider diversity and equity in AI research, both in terms of the research workforce and the research impact. NHMRC and HMRO could promote the inclusion of marginalised voices, address the potential biases and harms of AI, and ensure that AI does not widen the health gap.
Investment
- Focus on the health outcomes and the vision for AI in health, rather than the technology itself.
- Participants emphasised the importance of having relevant expertise and multi-disciplinary collaboration in AI research. They advised to consider:
- how selection criteria for NHMRC funding for people needs to be more accommodating of cross-disciplinary researchers, who are critical to harnessing the benefits of AI
- having multi-disciplinary peer review panels, with peer reviewers assessing different aspects of the grant
- how to support and incentivise multidisciplinary teams and collaborations across different domains and disciplines, and address the gaps and barriers between them
- how restrictions on the number of applications a person can be on in a specific scheme might impact on people with niche expertise who aim to provide a small input into several large projects
- how track record assessment in grant applications could disadvantage people from sectors other than health and medical research for example, ethicists, philosophers, technical experts
- developing a joint multidisciplinary funding call across NHMRC, HMRO and ARC to address the complex issues and opportunities of AI in health and medical research.
- Consider different models and mechanisms for funding AI research, such as targeted calls, missions, centres, or platforms.
- Benchmark Australia's investment and performance in AI research against international competitors and consider options for increasing support and incentives for multidisciplinary teams and translation.
- Invest in building the capacity and skills of researchers and health professionals to use AI effectively and ethically.
- Drive diversity in the research workforce through reporting on demographic characteristics of applicants and recipients, targeted grants for underrepresented groups, and finding ways to reflect more diverse views for developing grants.
Translation
- Provide guidance to applicants and assessors on essential elements of a translational project, to demonstrate that translation is feasible and worthwhile.
- Provide more funding for health services research to provide insight into how to solve implementation problems in a local context.
- Define what successful translation of health research using AI looks like and ways to measure or communicate the impact.
- Facilitate inclusion of industry and device manufacturers into translational research programs.
Ethics and integrity
- Review existing NHMRC documents (for example, National Statement on Ethical Conduct in Human Research, Code for the Responsible Conduct of Research) and identify where they might need some extra guidance or policy around the use of AI. Suggested topics that could be covered in extended guidance included:
- secondary use of data
- transparency and building trust in AI in healthcare
- informed consent
- considering what data has been used for the foundation models used for pre-training
- guidance on synthetic data, as when it is generated from human subjects, this should not obviate application of the National Statement on Ethical Conduct in Human Research
- potential bias in algorithms and the data used, for example, interrogating the integrity of the data input to ensure generalisability and that it is unbiased
- guidance on generative AI
- standards for governance, custodianship and management or evaluation of bias
- governance and data privacy guidance that defines stakeholder roles and ensures secure data handling
- ways to demonstrate transparency of AI use and its ‘black box’ nature, to improve quality and trust
- implications for merit where multi-stakeholder and -disciplinary expertise is engaged and stakeholders in some cases may be providing research data as quasi participants (for example, usability data, fidelity) and quasi-investigators (implementing tools)
- implications of potential for long range, indirect, or dual-use impacts particularly where initial validation may be conducted in one setting, without full implementation evaluation (this might also occur where, for example, a model is developed in one context and adopted into another)
- implication of commercial interests
- since many AI interventions will be adaptive then a 'certify once' model may not be appropriate, as the AI performance changes with time. There is a need for 'post-market' -like mechanisms that ensure ongoing compliance. This would address the need for reproducibility as the models will ‘drift’ and perhaps require a re-certification cycle
- whether research institutions have governance mechanisms that provide oversight of research that falls outside 'human research', and the potential for long range or implementation impacts that may not be considered by ethics committee process but that are important, and where ethics may not be well communicated in public outputs
- mitigating against marginalised voices becoming further marginalised through research about AI and/or research using AI.
- Collaborate with relevant bodies to establish clear guidelines about where responsibilities lie for clinical decisions made with assistance of AI.
Conclusion
NHMRC is grateful for the enthusiastic participation and valuable advice provided by the workshop participants. NHMRC will consider the information and suggestions in this report, in consultation with the HMRO and the joint NHMRC/HMRO advisory committees, when developing plans for next steps in the focus areas of investment, translation and ethics/integrity.
Appendix A - Background information provided to workshop participants
Australian government
The Australian Government has identified AI as a critical technology in the national interest. In 2019, the Department of Industry, Science and Resources (DISR) released The Artificial Intelligence Ethics Framework to guide businesses and governments to responsibly design, develop and implement AI. The Digital Transformation Agency and DISR have:
- released interim guidance on government use of publicly available generative AI platforms. The interim guidance is recommended for government agencies to use as the basis for providing generative AI guidance to their staff.
- established the Artificial Intelligence in Government Taskforce in September 2023. The Taskforce has representatives from agencies across the Australian Public Service (APS) and is focused on the safe and responsible use of AI by the APS
- consulted on the safe and responsible use of AI and published an interim response to the consultation.
Some other recent considerations of the ethics for use of AI include:
- World Health Organisation: Ethics and governance of artificial intelligence for health: guidance on large multi-modal models
- Nature: Living guidelines for generative AI
- Australian Alliance for Artificial Intelligence in Healthcare (AAAiH): A National Policy Roadmap for Artificial Intelligence in Healthcare
- Department of Industry, Science and Resources: Australia's AI ethics principles
- The Nuffield Council on Bioethics: AI in healthcare and research
- The Hastings Center: Artificial Intelligence Archives
- Bioethics Advisory Committee: Ethical, legal and social issues arising from big data and artificial intelligence use in human biomedical research
- Department of Industry, Science and Resources: Responsible AI Network
- UNESCO: Ethics of Artificial Intelligence.
International funding agencies
NHMRC is holding this workshop to obtain advice on the opportunities and risks from the use of artificial intelligence in NHMRC-funded health and medical research, including its impact on NHMRC grant processes and research translation to improve health outcomes. As guidance for workshop participants, below are some examples of what international funding agencies are doing to support health and medical research using AI.
UK Research and Innovation articulates its vision for and investments in AI - How we work in artificial intelligence.
UK Research and Innovation explored the opportunities presented by AI and how they could engage with and support AI research and innovation - Transforming our world with AI.
This news article from Wellcome provides a basic overview of AI in health and gives some broad suggestions for researchers using AI - How is AI reshaping health research.
Wellcome published a report exploring the application of AI in drug discovery, with some specific recommendations for funders - Unlocking the potential of AI in drug discovery.
The UK-based Research Funders Policy Group published a statement in September 2023 that:
- researchers must ensure generative AI tools are used responsibly and in accordance with relevant legal and ethical standards where these exist or as they develop
- any outputs from generative AI tools in funding applications should be acknowledged
- peer reviewers must not input content from confidential funding applications or reviews into, or use, generative AI tools to develop their peer review critiques or applicant responses to critiques.
The National Institutes of Health outlines their artificial intelligence activities - Artificial Intelligence at NIH. These include funding initiatives to:
- improve skills for making data findable, accessible, interoperable, reusable and AI-ready
- address ethics, bias and transparency
- make data generated through NIH-funded research AI-ready
- generate new 'flagship' data sets and best practices for machine learning analysis
- increase the participation and representation of researchers and communities currently underrepresented in the development of AI models
- develop ethically focused and data-driven multimodal AI approaches.
The use of generative AI technologies is prohibited for the NIH peer review process - NOT-OD-23-149: The Use of Generative Artificial Intelligence Technologies is Prohibited for the NIH Peer Review Process
Appendix B - NHMRC and HMRO’s previous investments in research involving or related to AI
Between 2015 and 2023, the two funds have funded:
- MRFF - $96.3 million, 35 grants
- MREA – awarded over $106.4 million, at least 100 grants.1
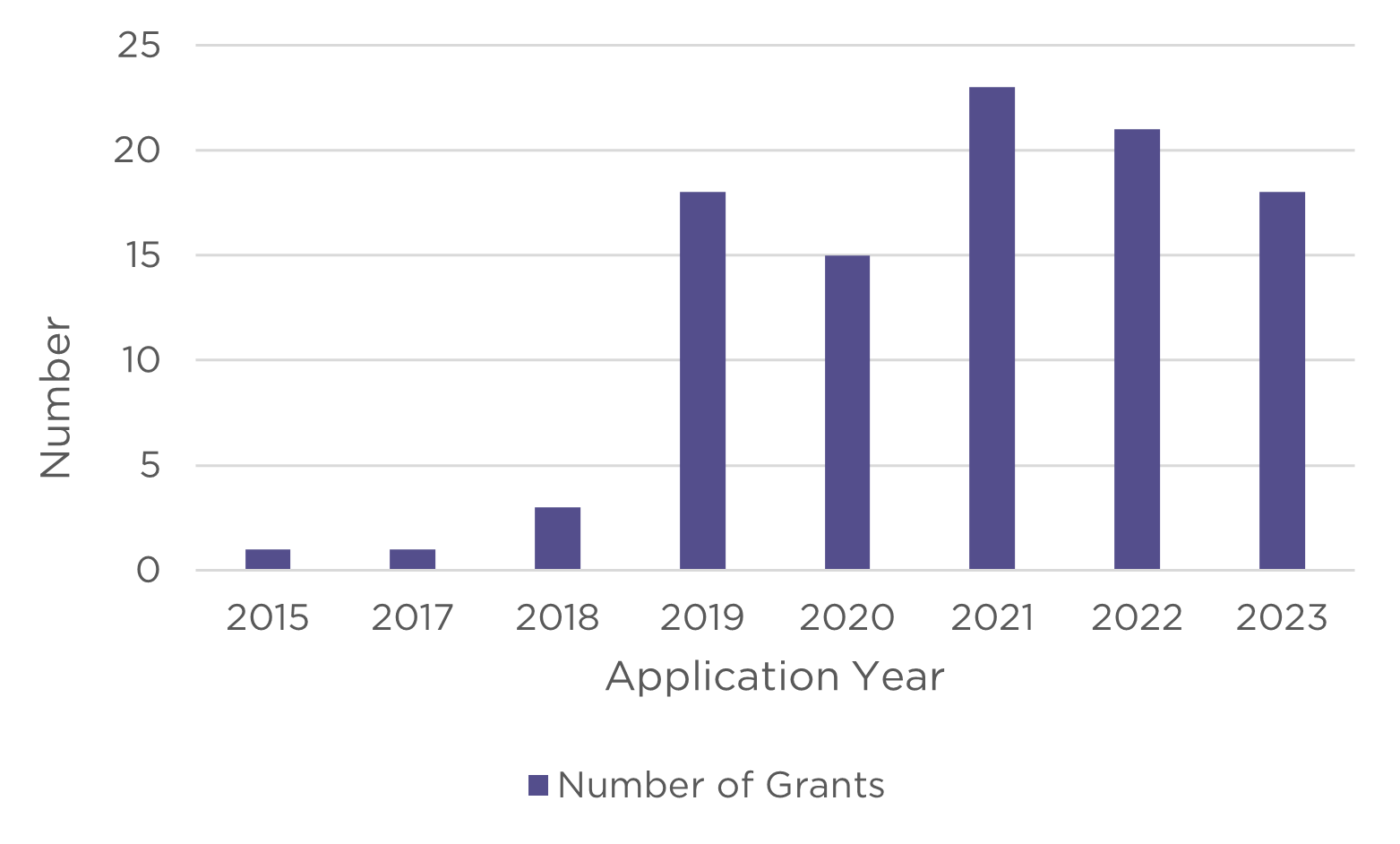
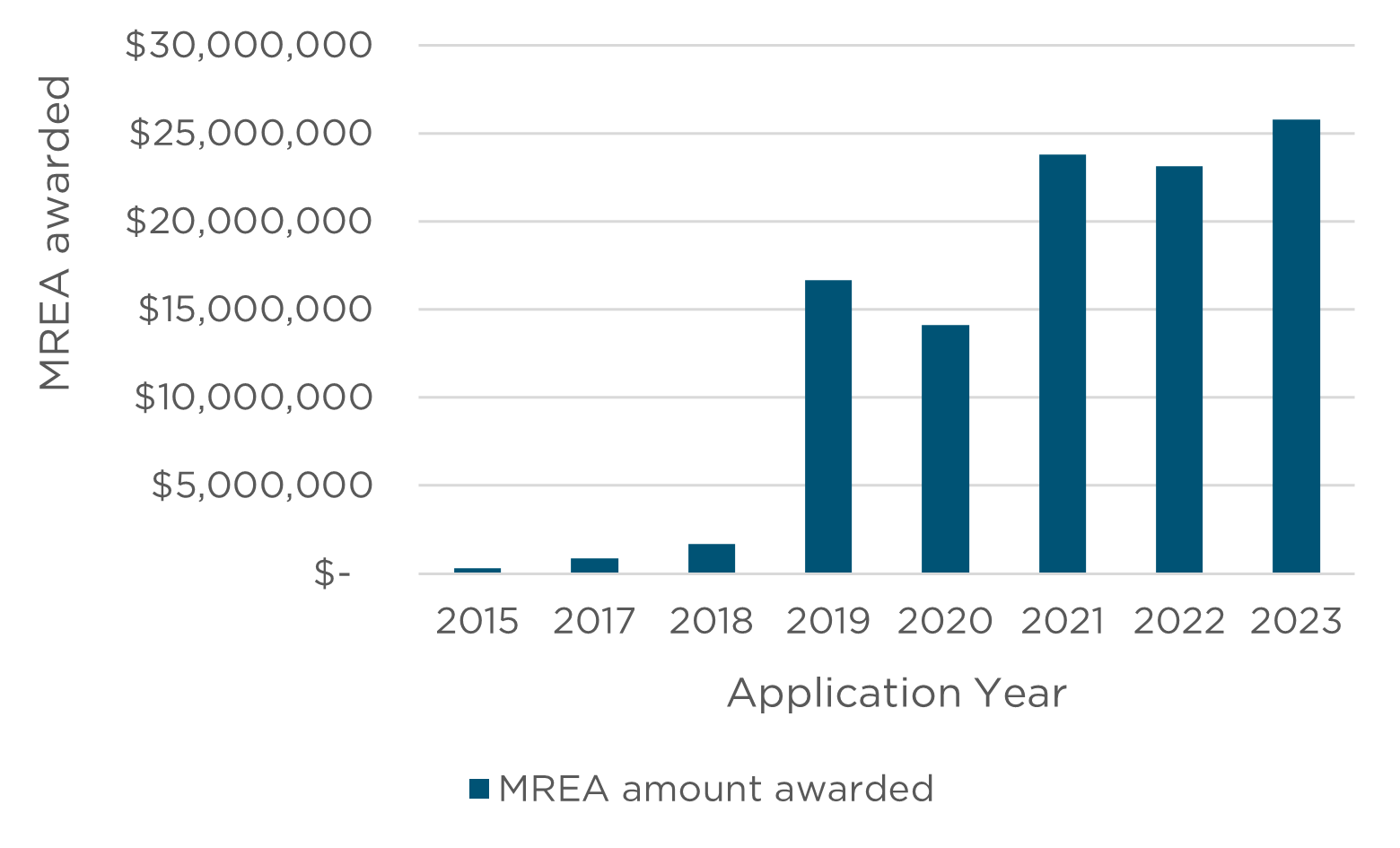
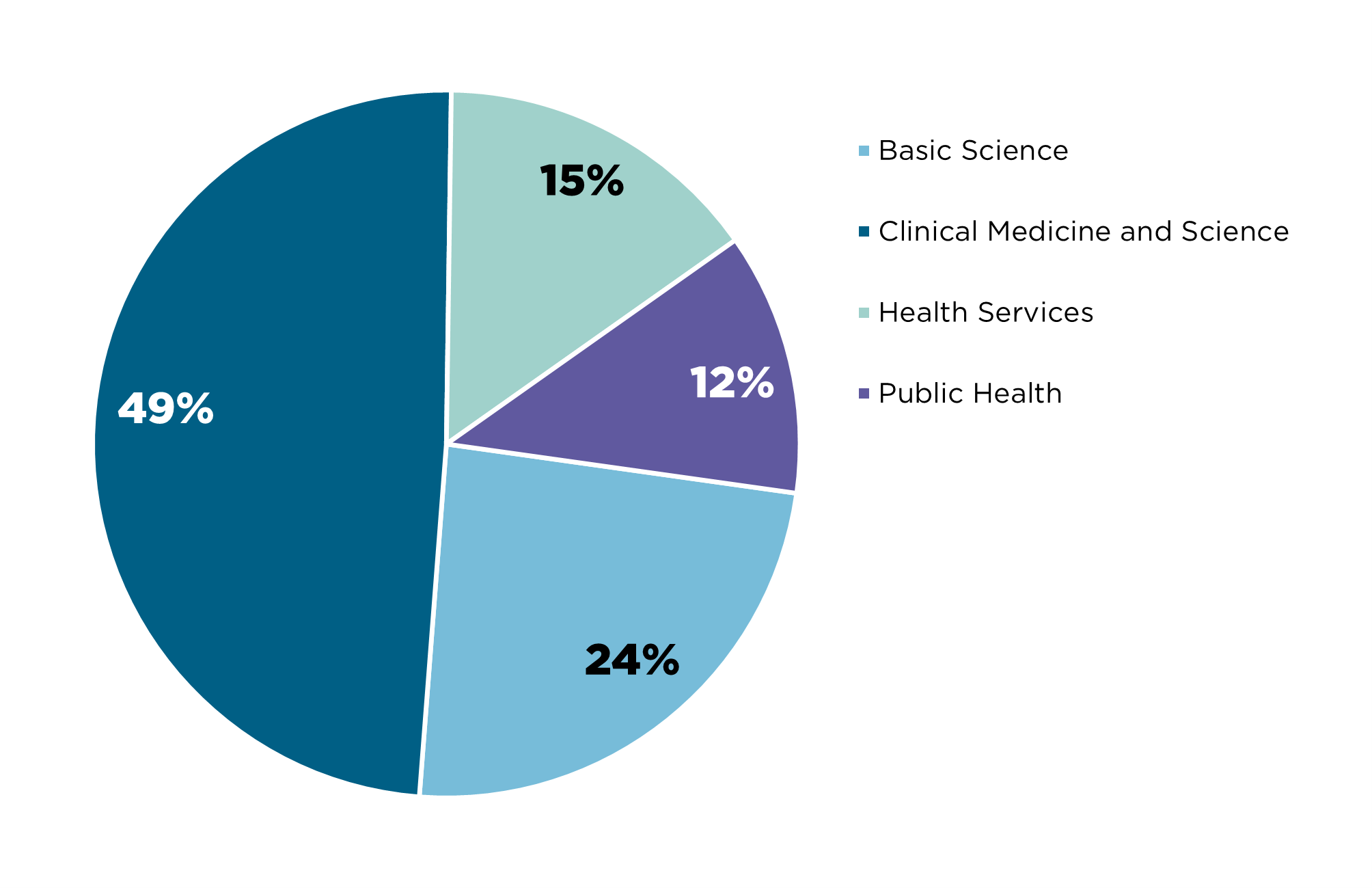
1 where the researcher has indicated that the research will either involve or be relevant to artificial intelligence as part of their grant title, keywords, fields of research or plain description